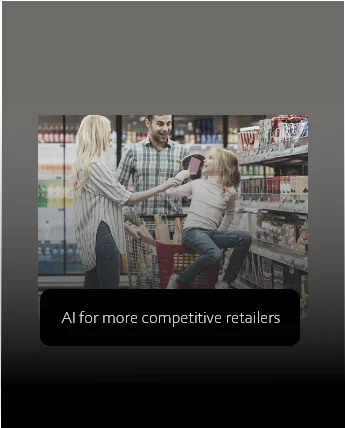
Autor:
Cristian Zorrilla – Interaction Designer Equinox AI Lab
AI IN RETAIL
As Artificial Intelligence capabilities expand, its solutions keep transforming the landscape of retail businesses across the spectrum.
From streamlining administrative tasks with automation to virtual shopping experiences created through real-time advertising, artificial intelligence has made it simpler to increase business output’s speed, efficiency, and accuracy. This enhanced performance is linked closely to advanced data and predictive analytics systems that help companies make data-driven business decisions.
Retailers are increasingly looking for ways to optimise their business by adapting to new trends, understanding new market strategies, and utilising new technologies. As a result, they are looking to integrate technologies such as artificial intelligence, data science, data analytics, and RPA (Robotic Process Automation) into their businesses.
RPA consists of automating processes with robots, following established rules, so these robots can perform repetitive tasks, such as:
- Entering and reviewing data.
- Pressing buttons.
- Uploading or downloading files.
- Making or paying invoices,
Automating these tasks will save you money and help your employees because they will have more time to focus on more critical tasks.
At least £216 billion of economic growth in the UK (representing 10.1% of the UK economy per year) was achieved thanks to technology. Of this, £54 billion was acquired by London, demonstrating the great power that many companies are already harnessing to boost their business.
AI allows you to evade manual errors, empower human capabilities, automate processes and provide accurate results. According to Forbes magazine, AI will be able to increase the workforce by 70% by 2025 (Gow, 2022). Also the industry is expected to increase its value by $36.8 billion by 2025, which changes consumer preferences and customer buying patterns, meaning a significant factor for industry growth in the following years.
AI also offers insight into consumers’ behavioural analytics, which retailers can utilise to gain insights to help enhance different touchpoints throughout the customer journey. Our mission at Equinox is to build a retail solution that caters to the individual needs of your business.
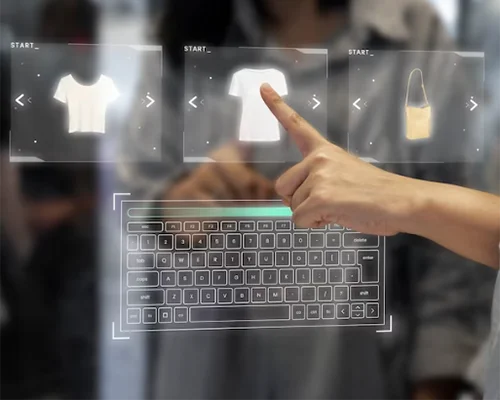
Equinox and its impact on retailers
At Equinox, we provide complete solutions, as we always seek to implement more than one technology when designing our projects. Therefore, all our solutions implement AI+Data+RPA because we will always give you a complete solution that intervenes in multiple problems.
With our team’s multidisciplinary work, we can address any need that our customers may bear since it comprises developers, designers, psychologists, anthropologists, AI engineers, data analysts, data scientists, and RPA specialists.
Better performance
Over time people have realised the almost infinite potential of Artificial Intelligence or AI for short, being an excellent tool when it comes to selling. For example, AI can learn about your customers so that you can learn about their preferences, behaviour and needs.
AI also allows you to create advanced algorithms to know what your customers might be interested in based on demographics, social media behaviour and buying patterns.
We can help you improve a lot of processes within your company, such as synchronising your physical and virtual store, which will allow you to know the status and behaviour of these channels at all times, being your virtual store a complement to your physical store and vice versa, which will allow these two channels do not overlap but work in harmony, complementing each other.
We can also optimise many internal processes, such as the supply chain, making it much more efficient and faster as we can track exactly where your supplies are at all times. On the other hand, we can use the RPA specifically for the supply chain.
In this way, the robots will be able to program shipping schedules. They can also know the state of supply and demand so they can notify you when a specific product is running out, or even better, they can order the product and pay automatically.
A study conducted by the IGS (Information Service Group) found that using RPA in the supply chain reduces approximately 43% of the resources implemented for this end-to-end process, which includes tasks such as invoicing, credits, collections, pricing and others (Pillai, 2021).
User understanding and assistance
Thanks to AI, RPA and Data tools, you will know exactly what your customers require to sell more, you will be able to understand the most optimal time to push your products, and you will even be able to provide them with the products your customers need before they even know they need them.
You will also be able to optimise the user experience of your customers because you will offer them exactly what they are looking for at a price they are willing to pay or, for example, create a relationship of loyalty with your customers because you will always have at hand what they expect when they need it.
Another vital AI tool is Machine Learning, this will allow you to find patterns in your customers’ purchases by analysing what they buy the most and when they buy it, which will enable you to recommend products they can buy together, for example, this is what Amazon is currently doing:
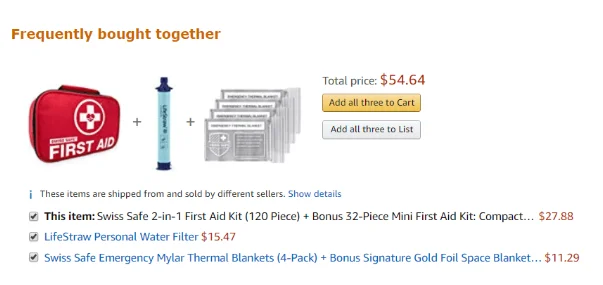
The image is taken from Google Images
AI could even recommend which products you should put together in your physical store, making you sell much more. AI has also empowered different businesses with high-level data, which has exponentially improved their internal operations and helped you find new business opportunities.
AI also allows you to create interactive chats or Chatbots. These can converse with your customers, allowing you to improve your customer service, as they can answer frequently asked questions, report on the status of their orders and provide them with the help they need 24/7.
In addition, these bots can collect information, so they can learn from your customers, helping you to have valuable information that will allow you to make better business decisions.
Image recognition and analysis is another excellent AI tool that helps your customers discover new or related products, allowing you to create recommendations based on the aesthetics and similarities of the products.
Internal processes
A problem that retailers have always had is the disconnect between their physical store and their online store, as they usually work in very different ways and have different approaches, causing your shoppers to feel an unpleasant shopping experience, leading to inefficient operations in both channels.
AI is an excellent solution since it can synchronise your different channels, giving you, for example, A list of your complete inventory and ways to take advantage of your online store and your physical store at the same time, allowing you to know what your shoppers prefer, whether to buy online or have the products in material, which will enable you to prioritise one channel, but of course without forgetting the potential of the other channels.
User experience
Now, we can also use AI for more experimental products, such as virtual fitting rooms that offer a much more personalised experience to your customers, as this allows them to quickly find the perfect outfit, plus your customers will be able to try on the different garments, without the need to put them on.
This is achieved thanks to augmented reality, in this way your customers will have the outfit on, without the need to have it, it could also change its colour, size or design simply by pressing a button, which dramatically streamlines the selection of items and customers can see an extensive catalogue without the need to search for them in the store.
*Luxury brands are exploring and implementing AI interactions in their stores; click here to read more.
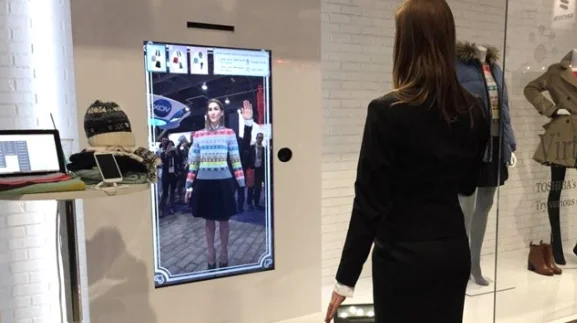
Image taken from Kid, A. R. (2018, 6 April). The virtual fitting room is the new trend in augmented reality. Augmented and Virtual Reality Company.
AI can also help you secure your shoppers’ data, recording the movements inside the store; it also allows you to encrypt their sensitive personal information, such as credit card information, personal data or shopping lists.
Also, thanks to video analytics or computer vision, you can check your physical store products in real-time and notice suspicious activities. This way would notify you of theft instantly, and you could take action as quickly as possible. AI will help prevent and alert you about theft or events in your stores.
CONCLUSION
AI, data analysis and RPA are practical tools for changing the retail industry, and we want you to be part of that change. Equinox AI Lab can be your guide in this process; if any of this information catches your attention, don’t hesitate to contact us or schedule a meeting with the help of our ChatBot.
REFERENCES
- Gow, G. (2022, 30 October). The Argument For An AI Augmented Workforce. Forbes. https://www.forbes.com/sites/glenngow/2022/10/30/the-argument-for-an-ai-augmented-workforce/?sh=87fbd7f2f4c6
- Kid, A. R. (2018, 6 April). Virtual fitting room, the new trend in augmented reality. Augmented and Virtual Reality Company. https://ardev.es/en/virtual-fitting-room-augmented-reality/
- How Artificial Intelligence (AI) Can Help Retail. (2019, 16 June). LiveAbout. https://www.liveabout.com/how-artificial-intelligence-will-change-retail-4143281
- AI In Retail: Uses Of Artificial Intelligence In Retail Business. (2022, 8 June). USM. https://usmsystems.com/what-artificial-intelligence-can-do-for-retail-industry/
- Digital britain: How small businesses are turning the tide on tech. (2022). Sage.
- Pillai, S. (2021, 22 septiembre). Why Supply Chain Agility Is Critical Today. Default. https://isg-one.com/articles/why-supply-chain-agility-is-critical-today?searchTerms=supply+chain
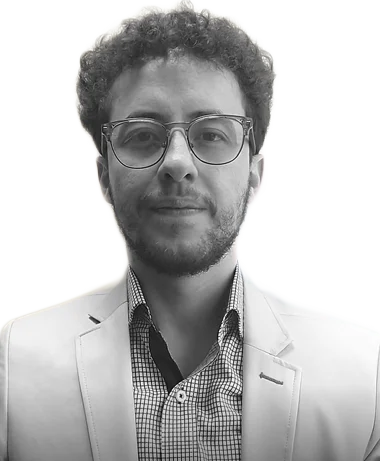
Cristian Zorrilla – Interaction Designer
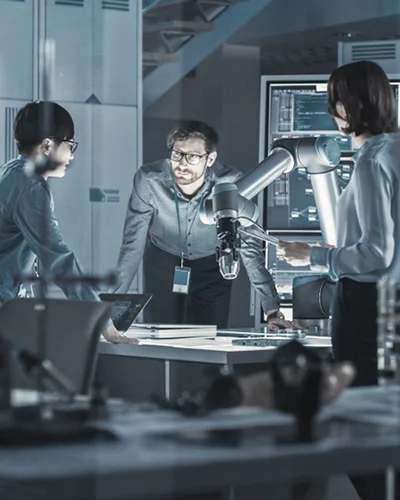
ARE YOU READY?
Ask for a free trial, request a demo, request pricing or simply ask any question!!