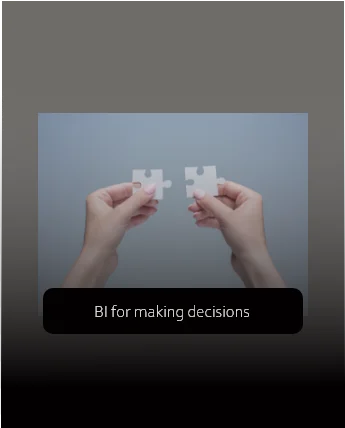
Autor:
Andrés Ruiz – Data Engineer
Making decisions has been decisive in the course of human history, and it is fundamental to demarcate what can happen in the future. This process is carried out in a constant way to solve from the simplest problem such as choosing which transport you want to use to get to your work faster, to even for more complex issues, for example, growing a company more.
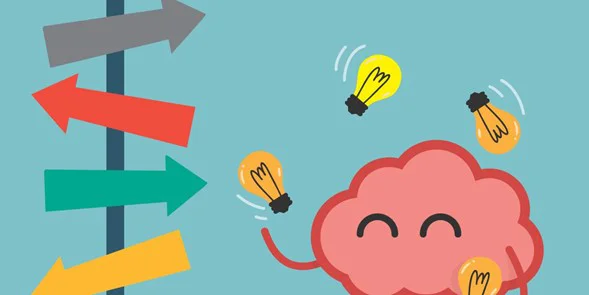
For this, the human being must realize a thought process that allows him to choose the best possible decision that can solve the problem, but in that case, would it be better to let ourselves be carried away by our intuition? Or is it better to do a complex process that allows us to analyze the problem and thus find the best alternative deeply?
It is clear that in order to solve a simple problem that is from our daily lives, it is enough to use our intuition, and surely we will be able to make a wise decision to solve the problem. Still, can this intuition be applied to solve more complex problems? The answer is that possibly the decision that is made will not be the most successful or the most efficient; it is here where Business Intelligence (BI) takes a fundamental role.
WHAT IS BI?
“Business Intelligence (BI) is an umbrella term for technology that enables data preparation, mining, management, and visualization. Business Intelligence tools and processes enable users to identify actionable insights from unprocessed data, making it easier to make data-driven decisions across companies across various industries.” IBM. What is BI? [1]
*Want to know first the relevance of data? Read our blog It´s all about data here.
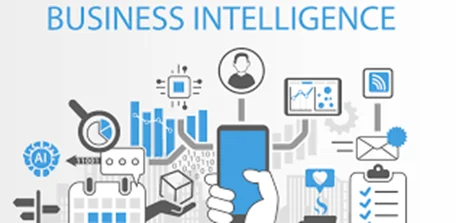
In other words, Business Intelligence is a set of tools that allows a person or organization to take advantage of a fundamental input such as data to generate “insights”. In a few words, information and knowledge can be extracted from the data, which serves as a basis to improve the decision-making process.
WHY USE BI?
BI offers organizations the opportunity to extract insights from data that serve as a basis for improving business decision-making. In addition, this allows organizations to operate in a more agile and efficient way by being able to find in the data the information they require to make intelligent decision-making related to any field of the organization [2].
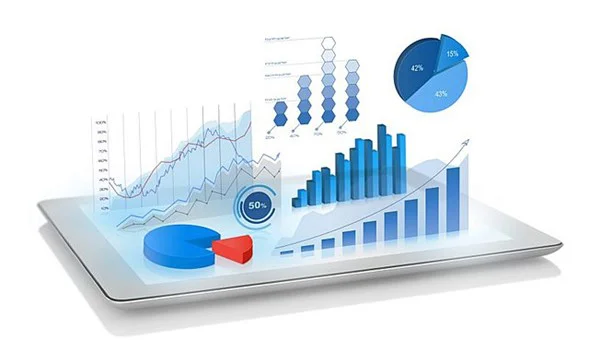
BI PROCESS
Applying Business Intelligence starts with analyzing the organization’s requirements and business questions. This first step is essential since it is necessary that the business questions and requirements are well defined to continue the process [3]. From this, you begin to follow the procedure shown in the following image.
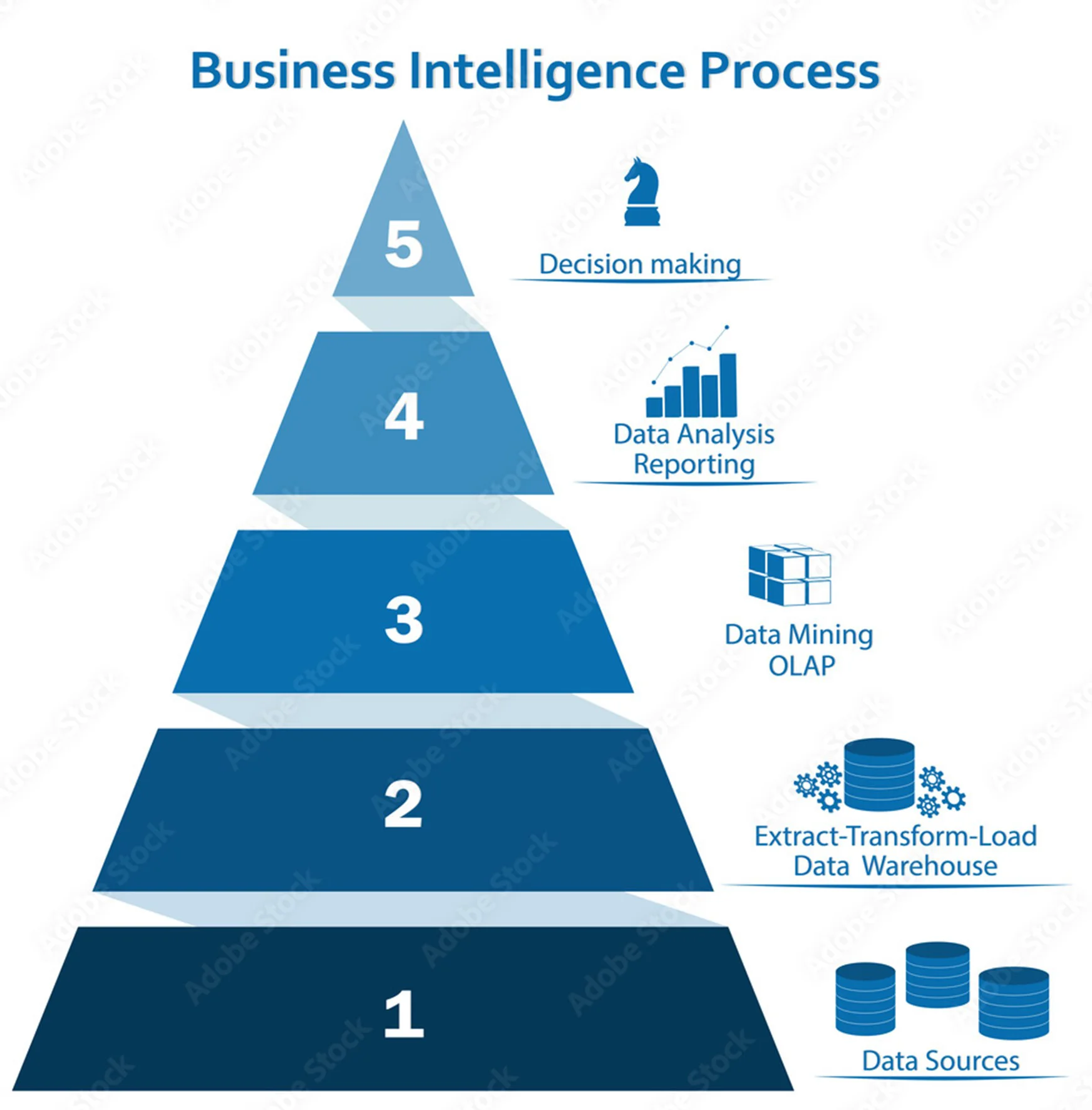
BI APPLICATIONS
- Data Sources: This step identifies the needed data sources to solve the requirements and business questions.
- Extract-Transform-Load DW: First of all, you must have the data model based on the requirements given by the organization. Subsequently, we proceed to carry out the ETL process from the Data Sources to the DWH.
- Data Mining: This process analyzes large quantities of data to find patterns such as groups of records, unusual records, and dependency [4].
- Data Analysis Reporting: In this step, data visualizations are used to allow us to find insights that enable us to respond to the requirements and business questions raised.
- Decision Making: This last step is where the relevant conclusions could be drawn to make way for intelligent decision making.
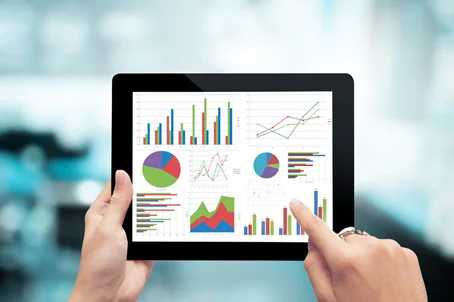
In conclusion, BI is important for organizations because it can help answer many business questions and for intelligent decisions that allow a relevant solution to a respective problem.
As seen above, BI could have different applications, all to improve aspects related to any area or field of the organization, which shows that it is currently an essential tool for the growth of organizations.
REFERENCES
[1] IBM. ¿Qué es la Inteligencia Empresarial. Recuperado 6 de Enero de 2022, de https://www.ibm.com/co-es/analytics/business-intelligence
[2] Tableau. ¿Qúe es la inteligencia de negocios? Guia sobre la inteligencia de negocios y por qué es importante. Recuperado 6 de Enero de 2022, de https://www.tableau.com/es-es/learn/articles/business-intelligence
[3] Sherman, R. (2015). Business Intelligence Guidebook From Data Integration to Analytics. Chapter 3, pág 44-45.
[4] Sherman, R. (2015). Business Intelligence Guidebook From Data Integration to Analytics. Chapter 1, pág 17.
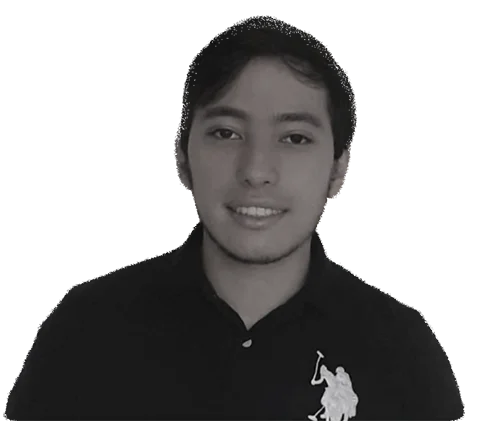
Andres Ruiz – Data Engineer

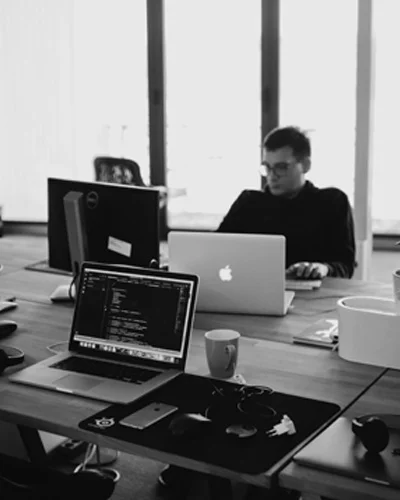
VISIT OUR KNOWLEDGE CENTER
We believe in democratized knowledge
Understanding for everyone: Infographics, blogs, and articles
Let’s tackle your business difficulties with technology
” There’s a big difference between impossible and hard to imagine. The first is about it; the second is about you “

Marvin Minsky, Professor pioneer in Artificial Intelligence