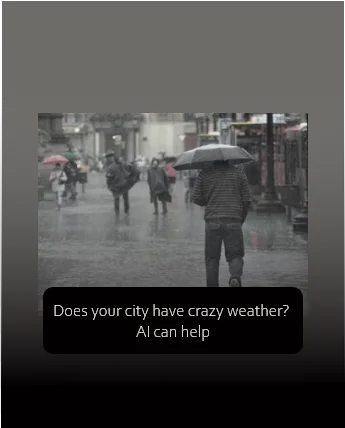
It is another beautiful day in your home city, and you see throughout your window up in the blue sky… no sign of rain the whole day. Having concluded that, you apply your sunscreen and decide to wear light clothes, and before going out, you wonder to yourself: ‘do I need to take out my umbrella? No, why would I put extra weight in my bag without the need of that.’
The day goes by, and just two hours after leaving
your home, in a blink of an eye, it starts to get cloudy and darker, and here comes the rain. The cities’ crazy weather is a fact that frustrates a big majority of its citizens each day; nonetheless, AI can come to the rescue by helping the weather forecast field in a short term period.
Forecasting the rain is not just helpful to save yourself from getting wet, but also to many industries that depend on the weather, like outdoor events, aerial services, tourism agencies, and farms, among others. The predictions with AI and deep learning methods are being tested for tornadoes’ and hurricanes’ formations as well; by this way, the people living under or close to the possible affected area can be informed on time to find refuge.
To have a context, in tornado-prone regions, the residents have, on average, an early alert of 16 minutes before the tornado impacts the zone [1]. However, this time range may not be enough for some people to find a safe place, and thus their lives can be in danger. For this specific case, with the data collected from humidity, air pressure, and temperature sensors during the previous minutes, with AI, the alert can go up to an hour before the tornado comes [1].
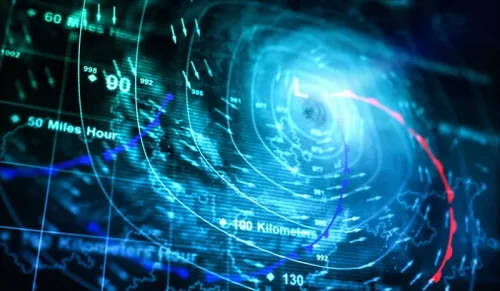
Taken from Analytics Insight: https://www.analyticsinsight.net/ai-empowering-weather-forecasting-technology/
Long-range and short-range forecasting
Now, let’s point out an important difference between two types of forecasting: long and short term.
Long-term forecasting refers to the prediction of weather for up to a month. This is done by mathematical and physical models aimed to simulate the physics of fluid dynamics weather [2]. These models usually are based on satellite images taken commonly in a year/month window. Because of the volatile and complex weather patterns, the aforementioned models are huge and take a lot of computer resources to be performed.
For short-term weather forecasting, also called nowcasting, the window size is reduced up to a few hours [3]. Here the mathematical models can become too complex due to the chaotic behavior of short climate periods. At this point, AI and deep learning are used to simplify the computational calculations and reduce the time at which these are performed.
Where to get the data to build the models?
There are a lot of sources from which scientists collect essential data to build the deep learning models, some more reliable and with more resolution than others. Some of the most used are:
–Radiosondes are balloons that measure atmospheric characteristics, such as temperature, pressure, and humidity as they move through the air. These radiosondes use a radio signal to communicate the data to a station [4].
–Radar stands for radio detection and ranging and sends out radio waves that bounce off the nearest object and return to a receiver. It can sense many precipitation characteristics such as location, motion, and intensity [4].
–Satellites are geostationary objects that rotate with the earth and can be split into three categories [4]:
–Visible satellites record storms, clouds, fires, and smog.
–Infrared satellites record clouds, water, land temperatures, and features on the ocean.
– Water vapor satellites look for the moisture content in the upper half of the atmosphere.
–Drones (Still in research) are a new technology used in this field to help with short-range forecasting. They are equipped with pressure, humidity, and temperature sensors that generate data sent to a base station.
Drones for weather forecasting
The use of drones in weather predictions is a young field of research that so far has shown promising results. These researches focus on the lowest layer of the earth’s atmosphere called the boundary layer, where most of the earth’s weather is happening.
Scientists have traditionally used weather balloons or weather stations to collect data and build the models; nonetheless, for the first tool, there’s the disadvantage of not being able to be fully controlled and depends on the wind direction. For the second method, these stations cannot be as high as needed, and they must be attached to a grounded object [1].
Drones have neither of these limitations, and they can fly with headwinds and even within a storm. However, because drones can provide higher resolution in terms of the sensed data, this issue creates challenges in the computational expense and the complexity of the physics parameterizations required in the forecasting models themselves [6].
One of the ongoing projects is directed by professor Philip Chilson at the University of Oklahoma. He envisions a drone network over a region equipped with a complete set of sensors. These non-tripulated vehicles will be launched hourly. Instead of causing more complex theoretical models, they will feed with data some AI methods [1].
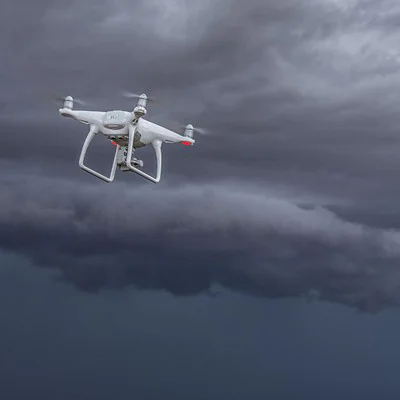
A model approach
The artificial intelligence company DeepMind, owned by Google-Parent-Alphabet, has the most advantage of research in weather prediction through AI. In the paper called “Skilful precipitation nowcasting using deep generative models of radar” [5], they found that 56 government meteorologists prefer the AI prediction model instead of other short-term forecasting methods in 89% of the cases.
The DeepMind model predicts up to two hours ahead thanks to weather data coming. The company developed a deep generative model (DGM), which is a statistical model that learns probability distributions of data and allows for the easy generation of samples from their learned distributions. The DGM is especially helpful to both learn from observational data as well as represent low uncertainty across a variety of spatial and temporal scales.
The DGM, in this case, is taken as probabilistic nowcasting of precipitation that addresses the almost random behavior of the climate. The methodology used helps to improve the current forecast quality and consistency through predictions over regions near to 1536 km x 1280 km with lead times from 5-90 minutes ahead.
These models are indicated to predict smaller-scale weather phenomena that are inherently hard to predict due to underlying stochasticity, which is critical for nowcasting. The input data to feed the model are radar-based observations of surface precipitation at a given time range and territory area. The mentioned observations keep updating the model at a frequency of 4 frames per 20 minutes. With this, the model is able to sample 18 frames of future precipitations in a period of 90 minutes [5].
The learning process step is driven by two loss functions and a regularization term, which guide parameter adjustment by comparing real radar observations to those generated by the model. The first loss is defined by a spatial discriminator, which is a convolutional neural network that aims to distinguish individual observed radar fields from generated fields, ensuring spatial consistency and discouraging blurry predictions.
The other loss is defined by a temporal discriminator, which is a three-dimensional convolutional neural network that aims to distinguish captured data from generated radar sequences; it imposes temporal consistency and penalizes jumpy predictions. Together with this, a regularization term is introduced to improve accuracy; this regularizer penalizes deviations at the grid search resolution between the real radar sequences and the model predictive mean. This feature also produces accurate location predictions and improves overall performance.
Speaking now about the training process, this model was prepared with a large dataset of precipitation events, which are 256 x 256 crops extracted from a radar source. The length is around 110 minutes with 22 frames. These images correspond to observations for the UK for years 2016-2018 and evaluated on a test set from 2019.
As a way of conclusion
Throughout all this article, we have been discussing how AI is helping the weather forecast and how this will help a lot of economic activities. With the guidance of high-resolution data from drones, for instance, the AI models can be fed with better sensed physical variables, and thus, they can give more concise results. The way until an exact tool for weather prediction is being walked with significant steps and soon whether to take out the umbrella or not will not be an issue anymore.
If you want to read more of our content follow the link : https://equinoxailab.ai/en/centro-de-conocimiento/
REFERENCIAS
[1] Elizabeth Ciobanu, “How drones are helping with weather forecasting” in Drone blog, Jan. 2022. [Online]. Available: https://www.droneblog.com/how-drones-are-helping-with-weather-forecasting/
[2] Aryan Thodupunuri, “The future of artificial intelligence in weather forecasting” in Towards AI, Aug. 2021. [Online]. Available: https://www.towardsai.net/p/l/the-future-of-artificial-intelligence-in-weather-forecasting
[3] James J., “How is long-range weather forecasting different than short-range forecasting?” in Socratic, Jul. 2015. [Online]. Available: https://socratic.org/questions/how-is-long-range-weather-forecasting-different-than-short-range-forecasting
[4] “Collecting weather data” in Lumen. [Online]. Available: https://courses.lumenlearning.com/geophysical/chapter/collecting-weather-data/
[5] Suman Ravuri, Karen Lenc et al., “Skilful precipitation nowcasting using deep generative models of radar” in Nature, Jul. 2021. [Online]. Available: https://www.nature.com/articles/s41586-021-03854-z.pdf
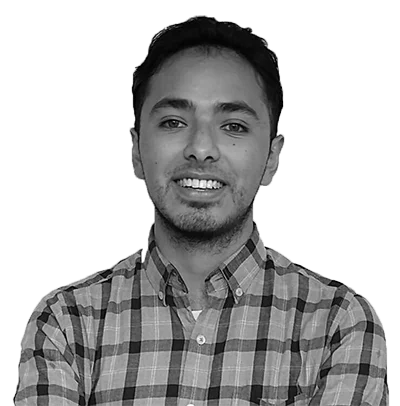
Favio Acosta – Data Scientist
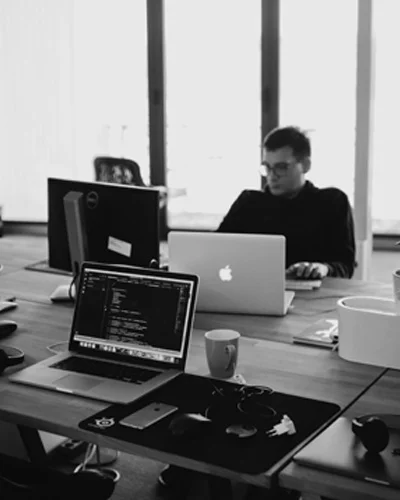
VISITA NUESTRO CENTRO DE CONOCIMIENTO
Creemos en el conocimiento democratizado
Conocimiento para todos: Infografías, blogs y artículos
Ataquemos las dificultades de tu negocio con tecnología
"Hay una gran diferencia entre lo imposible y lo difícil de imaginar. La primera tal vez lo es, la segunda se trata de ti"

Marvin Minsky, profesor pionero de la Inteligencia Artificial