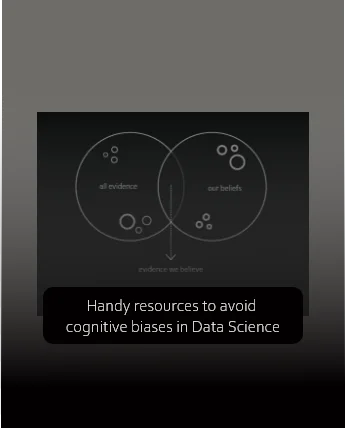
Author: Ivan Caballero – AI Designer
INTRODUCTION
If you were asked which animal is the world’s deadliest to humans, what animal would you say? Or if A bat and a ball cost $1.10, but the bat costs one dollar more than the ball. Do you know how much the ball costs? Or one that my father asked me once when I was a 10-year-old: what weighs more, a kilo of feathers or a kilo of potatoes?
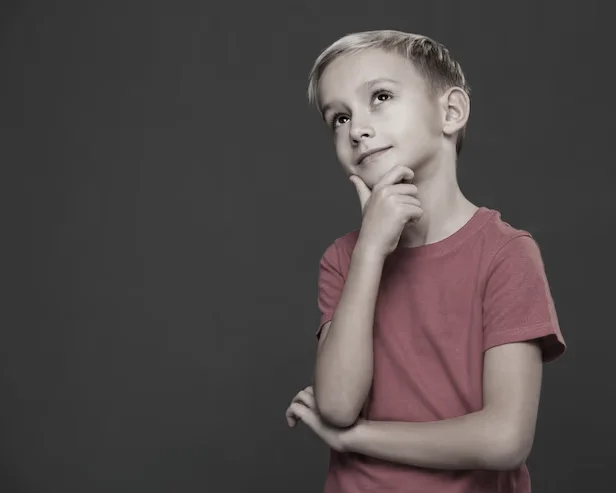
Perhaps you have heard or faced this type of tricky riddle that is usually used to make you fail in the answer and realise something: we struggle to be critical. Don’t worry; it is natural and inevitable in human beings. In trying to save energy and reduce cognitive load, our mind uses previous experiences to interpret information, make quick decisions, and have judgments about something. However, this process makes mistakes because many variables are overlooked, leaving us short of data and making incorrect judgments. This effect is known as cognitive bias.
In Ciencia de datos projects, it is crucial to consider the possible cognitive biases that teams face daily to know how we can avoid them because when understanding and processing a data set, building and designing artificial intelligence models or presenting information in graphs, these biases can affect the performance of what is built or present a completely distorted reality. That is why with this blog, we want to offer some online resources that can help you learn about the different varieties of biases and how to avoid them.
Wikipedia’s Cognitive Bias Codex
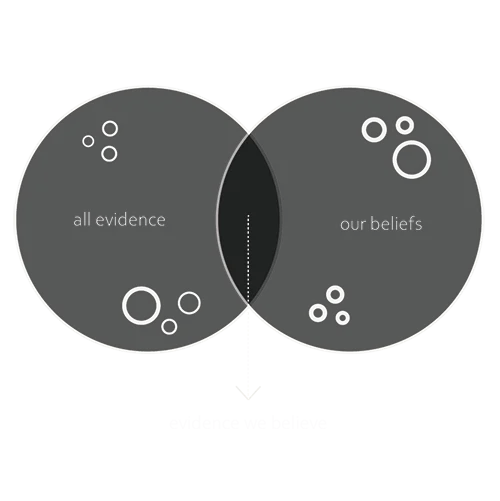
Confirmation bias diagram by Equinox AI Lab
https://commons.wikimedia.org/wiki/File:Cognitive_bias_codex_en.svg
This is a compilation of 188 cognitive biases categorised by Buster Benson, a marketing manager at Slack, and illustrated by user John Manoogian III (jm3) and linked to the articles written by user TilmannR on Wikipedia. Although for many, Wikipedia may not be a platform to trust; this graphic representation can help us have a quick reference guide to learn and keep in mind each of the biases and their leading causes.
Decision Lab list
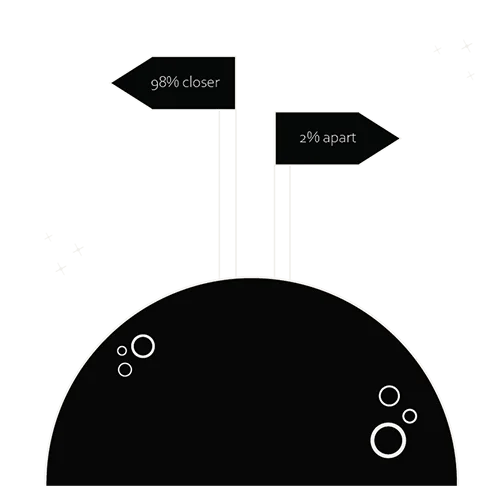
Framing Effect by Equinox AI Lab
This behavioural science firm has a list of biases categorised into ambiguity, information overload, memory, and speed. Each bias entry describes the bias, when and why it occurs, its effects when making decisions, how to prevent them, and daily life examples. This resource accurately explains biases, and its examples are easy to understand.
On the other hand, it is important for Data Science projects to present and analyse data effectively and realistically. Data visualisation should not be taken lightly. So here we also give you two key resources when it comes to avoiding biases in data visualisation.
From Data to Viz

Disposition Effect by Equinox AI Lab
https://www.data-to-viz.com/caveats.html
This website is intended to be a guide for designing accurate graphs according to what you need to represent in visualisation. Each bias entry is titled with the intention we have when plotting, the good and bad examples when using it and the considerations we must keep in mind to decide if the graph fits our intention.
Geckoboard
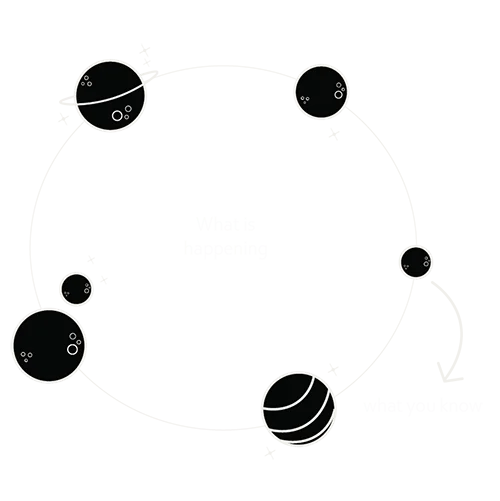
Availability bias by Equinox AI Lab
https://www.geckoboard.com/best-practice/statistical-fallacies/#.WsXXTmrFLIU
This company has a section where it lists 15 Data fallacies that may occur when interpreting and analysing data. There you will find what they are about, how to avoid them, and resources related to each fallacy.
Finally, you now have some resources you can take advantage of to avoid biases in Data Science projects. And if you were wondering what the answers to each of the riddles are, here are their solution: the deadliest animal for humans is the mosquito, the ball costs 5 cents, and to my 10-year-old self, both the feathers and the potatoes weigh a kilo :’).
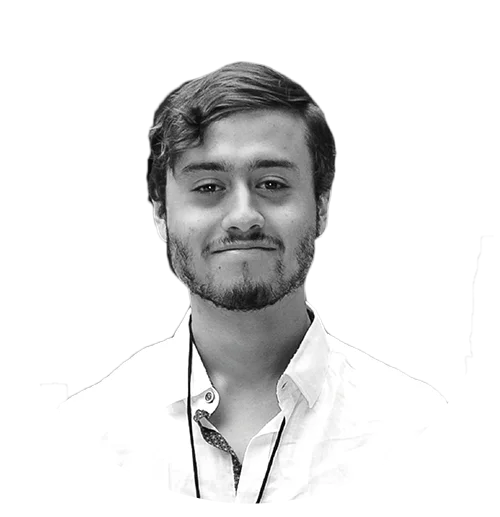
Ivan Caballero – AI Designer
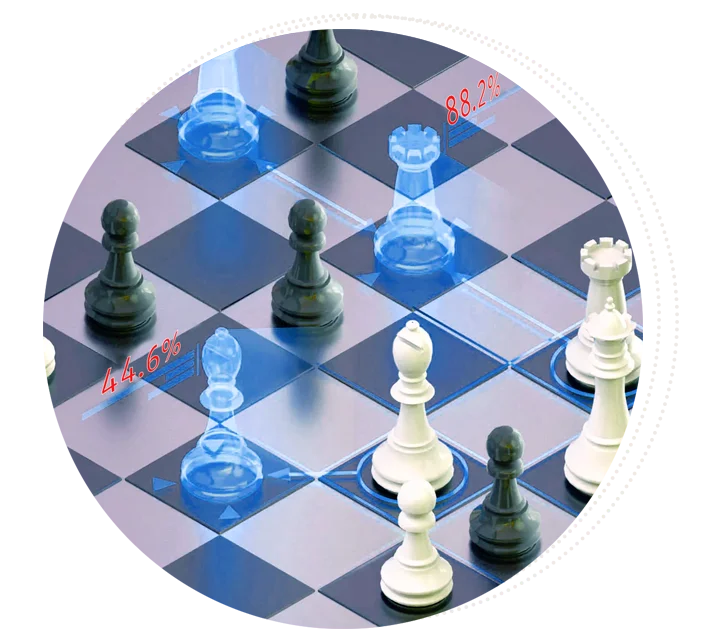