
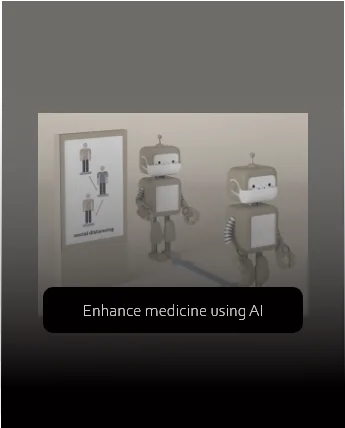
During the last decades, AI has transformed multiple fields of knowledge; medicine is not out of this transformation. There are many different ways in which we can enhance medicine using AI. In this article, I will introduce you to some of how AI can help discover new drugs, understand the mysteries of cancer, and learn up to one billion relations between different research resources.
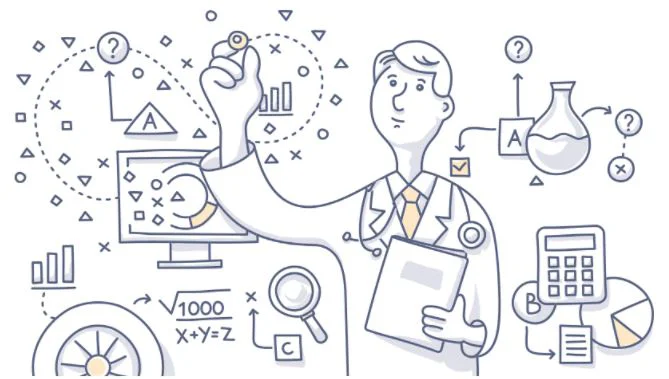
The first time AI helped humans research was in 2007 when Adam (a robot) generated hypotheses about which genes code for critical enzymes that catalyze reactions in the yeast Saccharomyces cerevisiae. Adam also used robotics to test its predictions in a lab physically. Researchers at the UK universities of Aberystwyth and Cambridge then independently tested Adam’sAdam’s hypotheses about the functions of 19 genes; 9 were new and accurate, and only one was wrong. [1] This is only one example of the multiple applications of AI in this field; get ready to learn more!
From understanding cancer to discovering new drugs
AI is turning the drug-discovery paradigm upside down by using patient-driven biology and data to derive more-predictive hypotheses rather than the traditional trial-and-error approach. For example, Boston’s Berg biotechnology company developed a model to identify previously unknown cancer mechanisms using tests on more than 1,000 cancerous and healthy human cell samples.
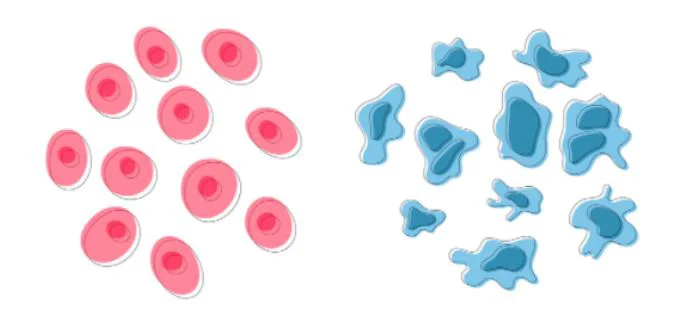
Another contribution of AI to this field was made by BenevolentBio when they created a platform that took information from multiple sources such as research papers, patents, clinical trials, and patient records.
Then this information is used to create a representation, based in the cloud, of more than one billion known and inferred relationships between biological entities such as genes, symptoms, diseases, proteins, tissues, species, and candidate drugs. The inference of those relationships uses Natural Language Processing (NLP) techniques.
Deepchem is a neural network model based on python used to find a suitable candidate in drug discovery aimed to democratize drug discovery, material science, quantum chemistry, and biology.[2] The Neural Network model used is a multilayer perceptron, a neural network where the mapping between inputs and outputs is non-linear. A Multilayer Perceptron has input and output layers, and one or more hidden layers with many neurons stacked together[3].
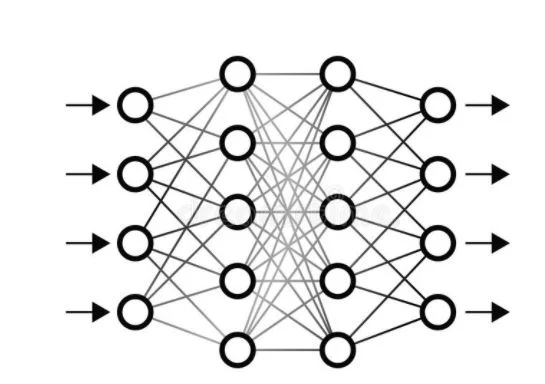
Uses and advantages
Only in the drug development field there are many applications of AI. In this article [4], the authors mapped the uses of AI in drug discovery:
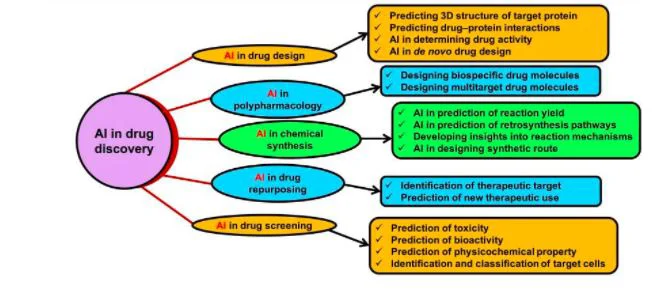
As you can see, there are a lot of applications in different fields, only in the drug discovery area. The prediction task has a central role in most of the uses. It is one of the preferred tasks of Artificial Intelligence!
Developing a new treatment can take over a decade and have an estimated cost of about 2.6-billion dollars. Much of that effectively goes down the drain because it includes money spent on the nine out of ten candidate therapies that fail somewhere between phase-1 trials and regulatory approval. With the right effort, AI and machine learning will usher in an era of optimizing quicker, cheaper, and more effective drug discovery. AI can recognize hit and lead compounds and provide a quicker validation of the drug target and optimize the drug structure design.[4]
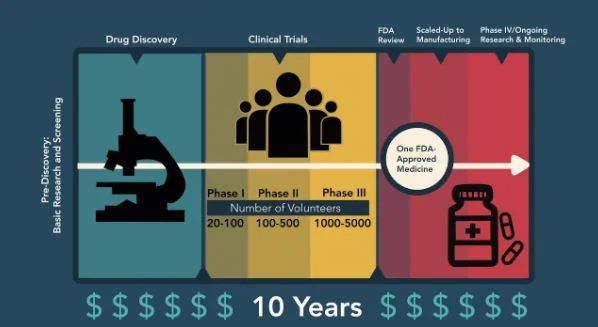
Some challenges
Despite these promising applications, many scientists are unaware of the capabilities of AI. A survey published in February by BenchSci, a start-up in Toronto, Canada, that provides a machine-learning tool for scientists searching for antibodies, found that 41% of the 330 drug-discovery researchers who took part were unfamiliar with the uses of AI [5].
AI faces some significant data challenges, such as the data’s scale, growth, diversity, and uncertainty. The data sets available for drug development in pharmaceutical companies can involve millions of compounds, and traditional ML tools might not be able to deal with these types of data. In addition, access to data from various database providers can incur extra costs to a company. The data should also be reliable and high quality to ensure accurate result prediction.
Other challenges that prevent full-fledged adoption of AI in the pharmaceutical industry include the lack of skilled personnel to operate AI-based platforms, limited budget for small organizations, apprehension of replacing humans leading to job loss, skepticism about the data generated by AI, and the black box phenomenon (i.e., how the AI platform reaches the conclusions)[4].
TO CONCLUDE
In spite of all the previously listed challenges, Artificial Intelligence will transform the world in the coming years. If we want to prepare, we have to endure each of these problems. Fortunately, AI is not stopping us from finding solutions and answers to different problems. If we look forward to the future, new tools, such as Computación Cuántica, will be available to develop chemical simulations [6]. If you are looking to enhance some processes in the pharmaceutical industry with AI help, don’t hesitate to contact us!
REFERENCIAS
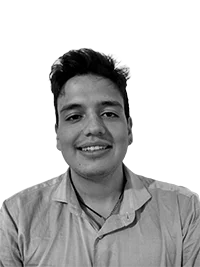
Daniel Panche – Data Scientist
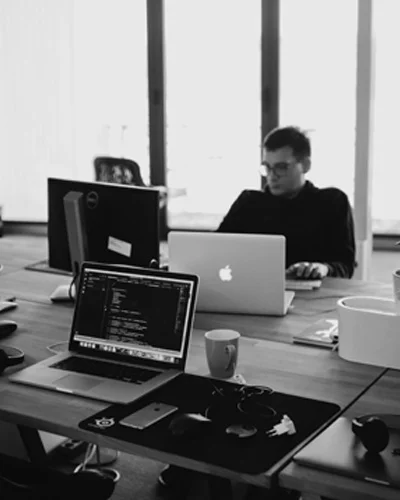
VISITA NUESTRO CENTRO DE CONOCIMIENTO
Creemos en el conocimiento democratizado
Conocimiento para todos: Infografías, blogs y artículos
Ataquemos las dificultades de tu negocio con tecnología
"Hay una gran diferencia entre lo imposible y lo difícil de imaginar. La primera tal vez lo es, la segunda se trata de ti"

Marvin Minsky, profesor pionero de la Inteligencia Artificial