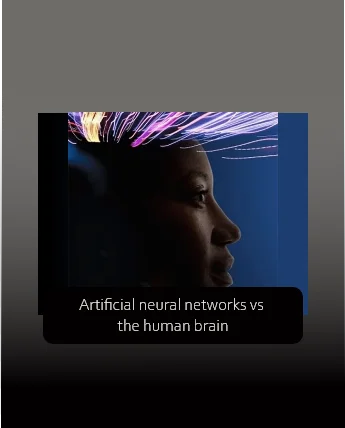
Author: Catherine Cabrera – Microbiologist, chemist and Data Scientist
¿Alguna vez se ha preguntado cómo aprendemos? Como científica, lo encuentro absolutamente fascinante. Todos y cada uno de nuestros pensamientos y acciones están controlados por uno de los órganos más increíbles de nuestro cuerpo: el cerebro. Y lo que es aún más alucinante es que todos los grandes logros de la humanidad a lo largo de la historia se basan en el sencillo, aunque complejo, proceso del aprendizaje. Observando la historia reciente, uno de estos asombrosos avances ha sido la inteligencia artificial (IA).
Hemos sido capaces de crear algunas entidades de IA realmente impresionantes que pueden hacer de todo, desde recrear imágenes y vídeos hasta jugar e incluso mantener conversaciones completas con nosotros. Es asombroso lo que podemos hacer con nuestro cerebro. La verdadera cuestión es si estas máquinas piensan o no. En caso afirmativo, ¿cómo lo hacen exactamente? Redes neuronales artificiales vs el cerebro humano investigará el interesante campo de la inteligencia artificial al tiempo que contrasta los procesos del pensamiento humano con estas increíbles entidades artificiales.
"Pienso luego existo"
"Pienso; luego, existo". René Descartes. Con esta sencilla frase, este filósofo fue capaz de captar la esencia del poder de nuestros pensamientos. Nuestra capacidad de pensar nos hace ser quienes somos: es lo que nos impulsa a hacer lo que hacemos, a sentir como sentimos y a conectar con los demás. En otras palabras, ¡todo lo que somos y todo lo que hacemos empieza con ese pequeño pensamiento en nuestra cabeza! Es increíble cuando te paras a pensarlo.
Aunque hay varias formas de aprender, el aprendizaje natural es una de las más sorprendentes. Podría interpretarse como el mecanismo mental que empleamos para captar información y habilidades sin un esfuerzo consciente. Nos permite aprender nuevas habilidades y llegar a nuevas conclusiones al permitirnos aplicar nuestras capacidades mentales, como la resolución de problemas, el pensamiento crítico y la toma de decisiones. También incluye diversas habilidades cognitivas como la percepción, la atención, la memoria y el procesamiento del lenguaje [1].
Desde un punto de vista biológico, nuestra capacidad única de pensar se debe a cómo interactúan las neuronas en nuestro cerebro. Cada neurona se compone de tres partes esenciales, un cuerpo celular, donde se almacena el material genético, y dos prolongaciones, un axón y una dendrita, que trabajan juntas para transmitir y recibir señales químicas a través de las neuronas mediante un proceso llamado sinapsis (fig. 1). La agregación de millones de neuronas es lo que hoy reconocemos como red neuronal natural (RNN) [2]. Alrededor de la década de 1950, el estudio del aprendizaje natural, la cognición y la arquitectura cerebral hizo surgir otra idea interesante: ¿podemos imitar el aprendizaje natural con un enfoque artificial?
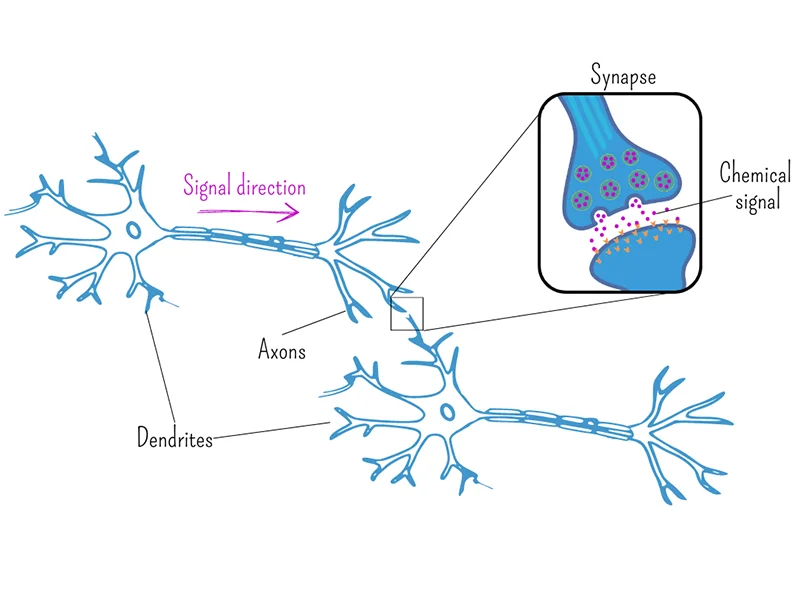
Fig. 1. Proceso de sinapsis y arquitectura neuronal en el cerebro humano. Realizado por Catherine Cabrera
Viajar a través de la historia - inteligencia artificial
Muchos pensadores a lo largo de la historia, como René Descartes y John Locke, debatieron la cuestión central de la conexión entre el cuerpo y el intelecto, sentando las bases para el desarrollo de la ciencia cognitiva [3]. Pero el campo de la inteligencia artificial no tomó forma hasta mediados del siglo XX, con la invención de los perceptrones. Se diseñaron para imitar la estructura y funcionalidad de las neuronas naturales y crear máquinas sencillas que pudieran resolver problemas de clasificación (Fig. 2).
La gente estaba muy entusiasmada con esta tecnología, ya que imaginaba todas las increíbles posibilidades de uso que podría tener. Por ejemplo, cuando la Marina trabajaba en 1958 en un ordenador electrónico basado en la tecnología del perceptrón, según un artículo del New York Times, no sólo sería capaz de andar, hablar y ver, sino también de escribir, reproducirse y ser consciente de su existencia [4].
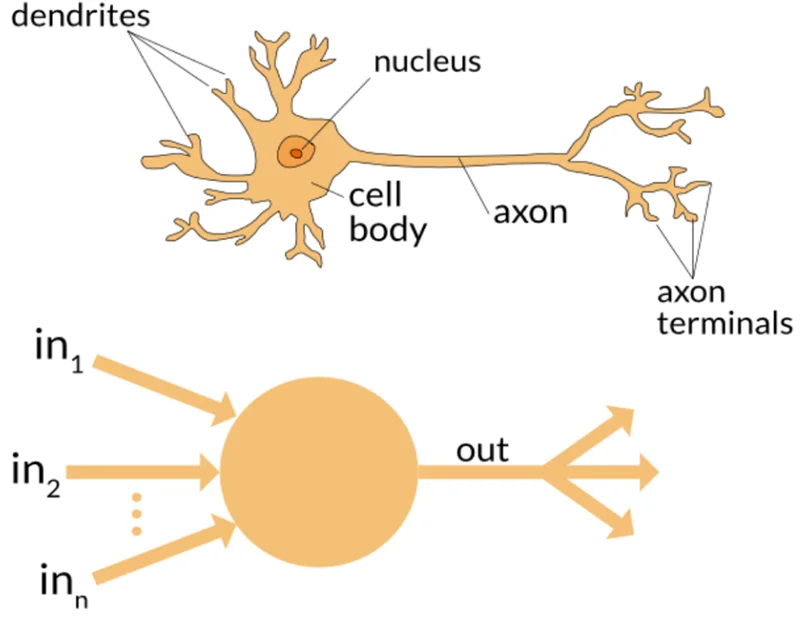
Fig. 2. Comparación entre perceptrones y neuronas biológicas. De: https://appliedgo.net/perceptron/
Y aunque los perceptrones eran capaces de tomar entradas, procesarlas y producir salidas, pronto se puso de manifiesto su limitada aplicación cuando fracasaron en la resolución de problemas no lineales como las funciones XOR [5]. Fue entonces cuando surgió la tentadora pregunta: si podemos imitar una sola neurona, ¿podríamos imitar toda una red neuronal? La relación entre el cerebro y la inteligencia desencadenó toda una nueva era de exploración, que condujo a los increíbles avances en IA que vemos hoy en día. Inicialmente, las redes neuronales artificiales (RNA) se hicieron uniendo múltiples capas de perceptrones simples, llegando a ser capaces de resolver problemas de reconocimiento de patrones; sin embargo, surgieron dos obstáculos; el entrenamiento de estas redes no era barato en términos de coste computacional y la respuesta binaria (0 o 1) que manejan los perceptrones, limita los problemas que estas redes neuronales pueden resolver [4].
Afortunadamente, hemos superado estos obstáculos y ahora podemos diseñar importantes entidades de inteligencia artificial como ChatGTP, You, Midjourney, Dalle-2 y otras. Este logro se consiguió con la creación de nuevas tecnologías y teorías revolucionarias que han cambiado por completo la arquitectura y la capacidad de procesamiento de las redes neuronales artificiales. Por ejemplo, se añadieron a las redes neuronales los procesos de recurrencia o retroalimentación de la información, lo que supuso un avance significativo en este campo y dio lugar a la creación de las redes neuronales recurrentes (RNN) (fig. 3). La instauración de este concepto en las RNA generó que estas redes comenzaran a divergir de las redes neuronales naturales, ya que no tenemos este proceso instaurado en nuestro cerebro. Basta considerar que en el proceso de sinapsis, la dirección de la señal es unidireccional [2].
Sin embargo, gracias a estas adaptaciones, ahora podemos ejecutar diversas tareas, como las de predicción de pruebas y las de síntesis del lenguaje. No obstante, las RNN también tienen ciertos inconvenientes, como su escasa memoria a corto plazo y sus complicadas curvas de aprendizaje. Las redes de memoria a corto plazo (LSTM) se desarrollaron para resolver estos problemas. Han cambiado por completo las reglas del juego al permitir a las RNN ampliar su memoria y realizar tareas que necesitan memoria a largo plazo (fig. 3). En una historia más reciente, se habían creado las redes neuronales convolucionales (CNN), una configuración tridimensional de neuronas artificiales, para tareas cada vez más complejas. Estas redes se emplean principalmente para actividades relacionadas con la visión por ordenador análogas a las que se pueden realizar con la propia vista (fig. 3) [5].
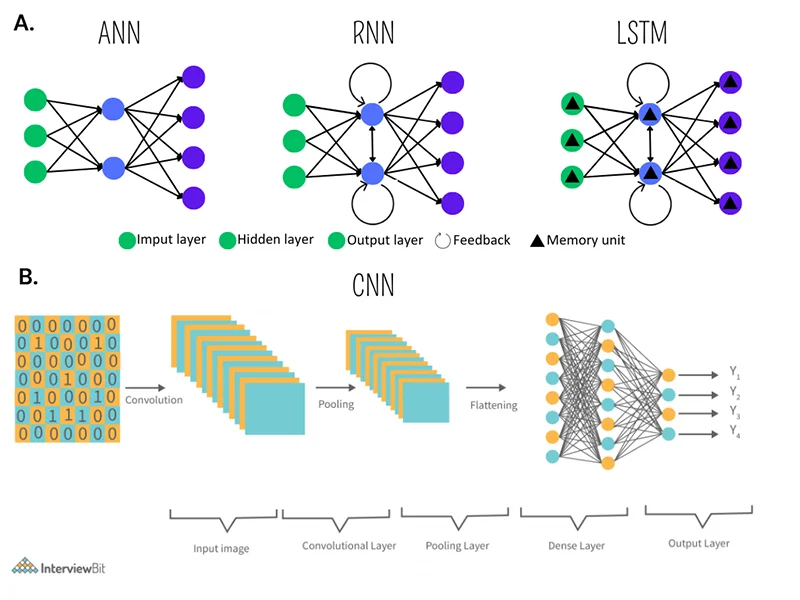
Fig. 3. Arquitectura de diferentes redes neuronales. Arquitectura de diferentes redes neuronales. a) Estructuras de ANN, RNN y LSTM. Realizado por Catherine Cabrera. b) Estructura básica de CNN, de https://www.interviewbit.com/blog/cnn-architecture/
Es fascinante cómo nuestra comprensión de las funciones cerebrales ha influido en la creación de la IA. Sin embargo, a pesar de inspirarse históricamente en el cerebro humano, la IA siempre se ha diseñado para funcionar de forma diferente a nosotros. Es como la diferencia entre un pájaro y un avión: ambos pueden volar, pero lo hacen a su manera. Del mismo modo, la IA tiene su propia forma de procesar la información y tomar decisiones, lo que la diferencia de nuestros procesos mentales. En concreto, el desarrollo de la IA se ha centrado en los datos. Además de combinar ciencia y tecnología para desarrollar estas máquinas, tenemos que dar datos a estas IA para enseñarles a "ver". Por ejemplo, al igual que una neurona biológica tiene dendritas para recibir señales, un cuerpo celular para procesarlas y un axón para transmitir mensajes a otras neuronas, para que un perceptrón haga una predicción se necesitan canales de entrada, una etapa de procesamiento y un canal de salida para entender los datos (fig. 2). En nuestro caso, las señales que recibimos son estímulos del mundo exterior, que posteriormente convertimos en señales químicas que el cerebro puede procesar. Para la IA, los datos son esa señal de entrada que necesitan para funcionar [1,6].
"A pesar de inspirarse históricamente en nuestro cerebro humano, la IA siempre se ha diseñado para funcionar de forma diferente a nosotros".
Catherine Cabrera – Química y microbióloga
¿Puede la IA pensar más que el cerebro humano?
Hoy en día, tanto el miedo como la curiosidad por las nuevas tecnologías de IA provocan un típico concepto erróneo sobre los puntos fuertes y las limitaciones que tienen. Por ejemplo, observar a una IA generar nuevas imágenes a partir de texto plano, reanudar grandes cantidades de información y crear vídeos, todo ello en cuestión de segundos, asusta, básicamente porque nosotros mismos no somos capaces de ello. Pero, ¿sabía que usted, o incluso un niño, probablemente pueda ganar al tres en raya contra algunas de las IA más asombrosas? Y aunque las redes neuronales artificiales se inspiraron en la función de nuestro cerebro, lo que las hace en cierto modo similares en concepto, importantes diferencias en estructura y capacidades de procesamiento provocan divergencias significativas en la funcionalidad que tienen, como se muestra en la siguiente tabla.
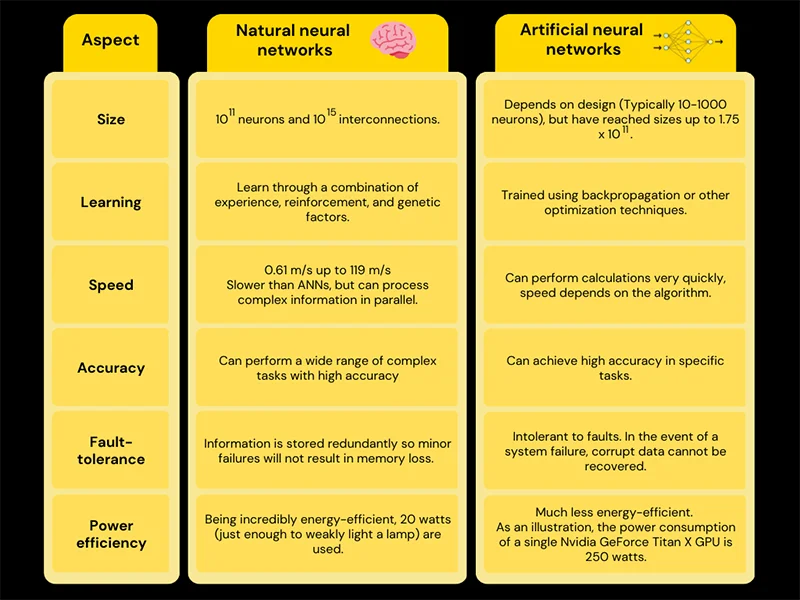
Tabla 1. Comparación entre redes neuronales naturales y redes neuronales artificiales. Realizado por Catherine Cabrera [4, 7].
A partir de la información expuesta anteriormente, podemos observar que existen algunas diferencias enormes entre las NNN y las RNA, como el tamaño, la flexibilidad y la eficiencia energética, entre otras. Sin embargo, comparar sólo números preliminares o aspectos concretos no es suficiente para entender el panorama completo, ya que el aprendizaje es un proceso que va más allá de la suma de estos aspectos.
Por ejemplo, ¿ha pensado alguna vez en lo distintas que son las máquinas de las personas en cuanto a la forma en que perciben e interpretan el mundo que las rodea? Es similar a equiparar sabiduría con conocimiento. A pesar de tener una gran cantidad de conocimientos en su memoria, la IA carece de la sabiduría necesaria para evaluar circunstancias complicadas que los humanos pueden manejar fácilmente. Por ejemplo, para nosotros puede ser sencillo identificar una imagen borrosa de un animal, pero para un sistema de IA puede ser difícil hacerlo debido a la rígida visión por computadora parámetros de entrenamiento que se utilizan para crearlas [8]. Por lo tanto, la próxima vez que te asombres del increíble talento de las IA, recuerda que hay cosas que solo los humanos son capaces de hacer.
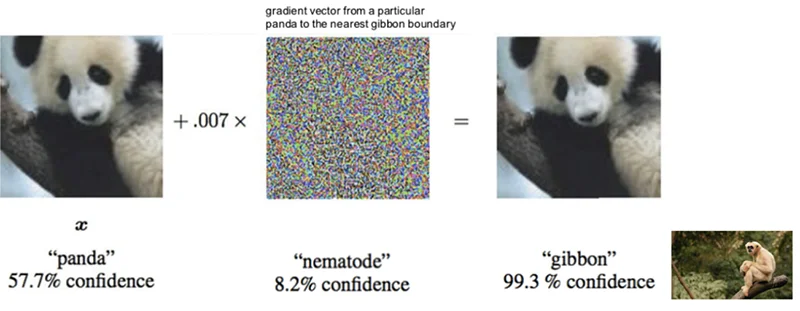
Fig. 4. Clasificación de imágenes realizada por la IA. En https://arxiv.org/abs/1412.6572)
Explorando esta idea, las IA son reconocidas por realizar tareas específicas con facilidad, pero ¿sabías que nuestra capacidad multitarea es una de las cosas que hace que nuestro cerebro sea tan increíble? Nuestra capacidad multitarea se debe a la naturaleza asíncrona y paralela de nuestras neuronas. Lamentablemente, la inteligencia artificial (IA) no puede igualar completamente nuestras capacidades en este sentido porque sus capas de neuronas artificiales suelen estar totalmente conectadas. Las neuronas artificiales necesitan pesos de cero para representar la falta de conexiones, en contraste con las neuronas biológicas, que son de mundo pequeño por naturaleza y pueden tener menos conexiones entre ellas [2,4,7]. Esto demuestra que, aunque la inteligencia artificial ha progresado mucho, la naturaleza sigue realizando algunas tareas mejor que la maquinaria más sofisticada. Además, los estrictos parámetros utilizados para construir las IA y su dependencia de los datos las hacen extremadamente vulnerables a cualquier fallo de software o hardware. En comparación, incluso si un segmento de nuestro cerebro sufre algún daño, podría seguir funcionando, ¡manteniéndonos con vida!
"Aunque la inteligencia artificial ha hecho grandes avances, la naturaleza sigue destacando en algunas tareas mejor incluso que la maquinaria más sofisticada".
Catherine Cabrera – Química y microbióloga
Conclusiones
A pesar de sus diferencias, el pensamiento natural y la IA pueden complementarse de muchas maneras. Por ejemplo, los sistemas de IA pueden utilizarse para ayudar al ser humano a tomar decisiones, aportando ideas y predicciones basadas en grandes cantidades de datos. A su vez, la cognición humana puede utilizarse para validar y refinar los resultados de los sistemas de IA, así como para proporcionar contexto e interpretar los resultados. Así que la próxima vez que aprendas algo nuevo, tómate un momento para maravillarte ante el increíble poder de tu cerebro y del aprendizaje natural, ¡y no te subestimes! No eres una máquina y no tienes por qué serlo.
REFERENCIAS
- Criss, E. (2008). The Natural Learning Process. Music Educators Journal, 95(2), 42–46. https://doi.org/10.1177/0027432108325071
- Brain Basics: The Life and Death of a Neuron. (2022). National Institute of Neurological Disorders and Stroke. https://www.ninds.nih.gov/health-information/public-education/brain-basics/brain-basics-life-and-death-neuron
- Gardner, H. (1987). The mind’s new science: A history of the cognitive revolution. Basic Books.
- Nagyfi, R. (2018). The differences between Artificial and Biological Neural Networks. Medium. https://towardsdatascience.com/the-differences-between-artificial-and-biological-neural-networks-a8b46db828b7
- 3 types of neural networks that AI uses. (2019). Artificial Intelligence|. https://www.allerin.com/blog/3-types-of-neural-networks-that-ai-uses
- Sejnowski, Terrence J. (2018). The Deep Learning Revolution. MIT Press. p. 47. ISBN 978-0-262-03803-4.
- Thakur, A. (2021). Fundamentals of Neural Networks. International Journal for Research in Applied Science and Engineering Technology, 9(VIII), 407–426. https://doi.org/10.22214/ijraset.2021.37362
- Ian J.et al. (2015). Explaining and Harnessing Adversarial Examples. Published as a conference paper at ICLR 2015. https://doi.org/10.48550/arXiv.1412.657
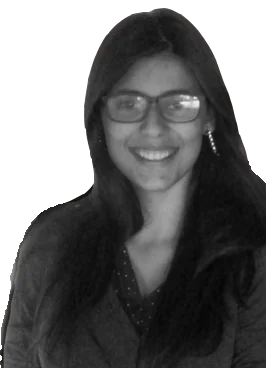
Catherine Cabrera – Data Scientist
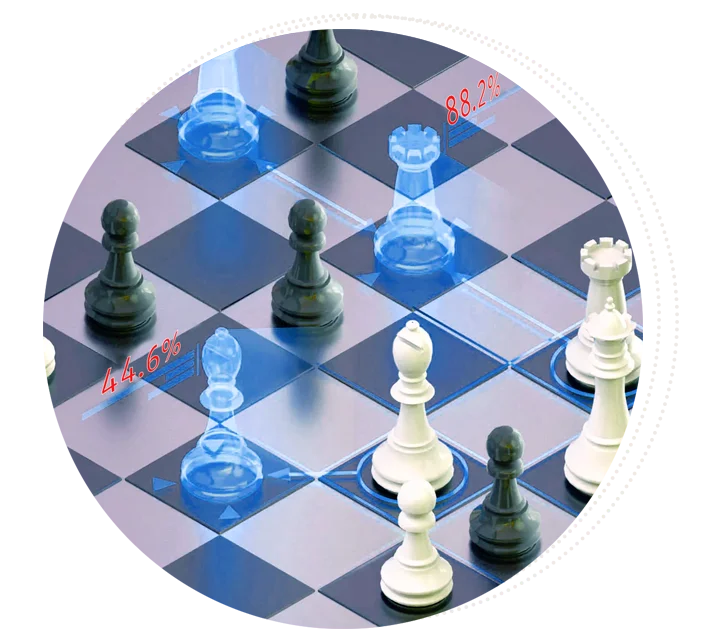